Research in the Noskov lab explores problems in protein biophysics and big data of drugs using computational approaches. Our focus is understanding ion channels and ion-coupled transporters, developing computational methods and using machine learning to predict side effects of pharmaceutical drug
Electronic Polarization and Metalloproteins
Modelling Electronic Effects in Metalloproteins
An importance of electronic degrees of freedom in biological processes is generally recognized in terms of enzymatic catalysis, where bonds are formed or broken. Recently, the critical role of polarization and charge transfer dynamics was proposed for proton wires, primary and secondary transporters and carbon nanotubes with implications ranging from selective ion transport in neurons (key for action potential) to design of desalination devices and nanosensors [Zhekova et al, 2017]. The utility of widely used QM or QM -MM minimization strategies can be limited in studies aimed at the modelling of membrane transport. The system size, complex dielectric environment and apparent need to exhaustive sampling for critical degrees of freedom from the protein, membrane, solvent and ion are all formidable challenges to QM calculations. To circumvent these limitation Noskov lab have contributed to the development novel sampling techniques and contributed extensively to the development of next-generation of potential models with explicit account for electronic polarization (Drude polarizable force-field for metalloproteins) [Ngo et al, 2015 and Wheatley et al, 2015].
Parameterization of Classical Drude Polarizable Force Field
Classical force-field models for molecular dynamics (MD) simulations, such as that depicted in Fig.A, require extensive parametrization in order to be consistent with both quantum predictions, as well as experimental data on macroscopic properties (such as solvation free energy). However, explicit account of polarizability is critical for descriptions of regions with high charge-density [Harder et al, 2015], such as binding sites for ions and/or charged ligands. The Drude polarizable model (Fig.B) is one such model which adds a virtual Drude particle to all atoms of the CHARMM36 force-field to account for polarizability to a dipole approximation. The current research in the lab focuses on ways to fit this model in order to reproduce both high-level QM data obtained to reproduce ion-metalloprotein interactions in a gas-phase [Li et al. 2015] and structural, thermodynamic and transport properties of the metalloproteins in a condensed phase. Fig.C illustrates the performance of this two-stage approach with the re-optimized Lennard Jones parameter for potassium ion and acetate interaction reproducing QM interaction energies in the gas-phase with classical Drude model. However, the direct applicability of QM-driven force-field is problematic [Ngo et al, 2019] and the further refinement of the parameter sets are warranted. The second stage involves iterative optimization of new parameter sets with osmotic pressure calculations over a range of concentrations. Fig.D features concentration-dependence of the osmotic pressure evaluated for the acetate-potassium aqueous salt solution. The project is a collaborative activity with Dennis R. Salahub (Calgary), Alexander MacKerell (UMD), Benoit Roux (Chicago) and Justin Lemkul (Virginia Tech) labs.
Mechanisms of Membrane Transport: Methods and Applications
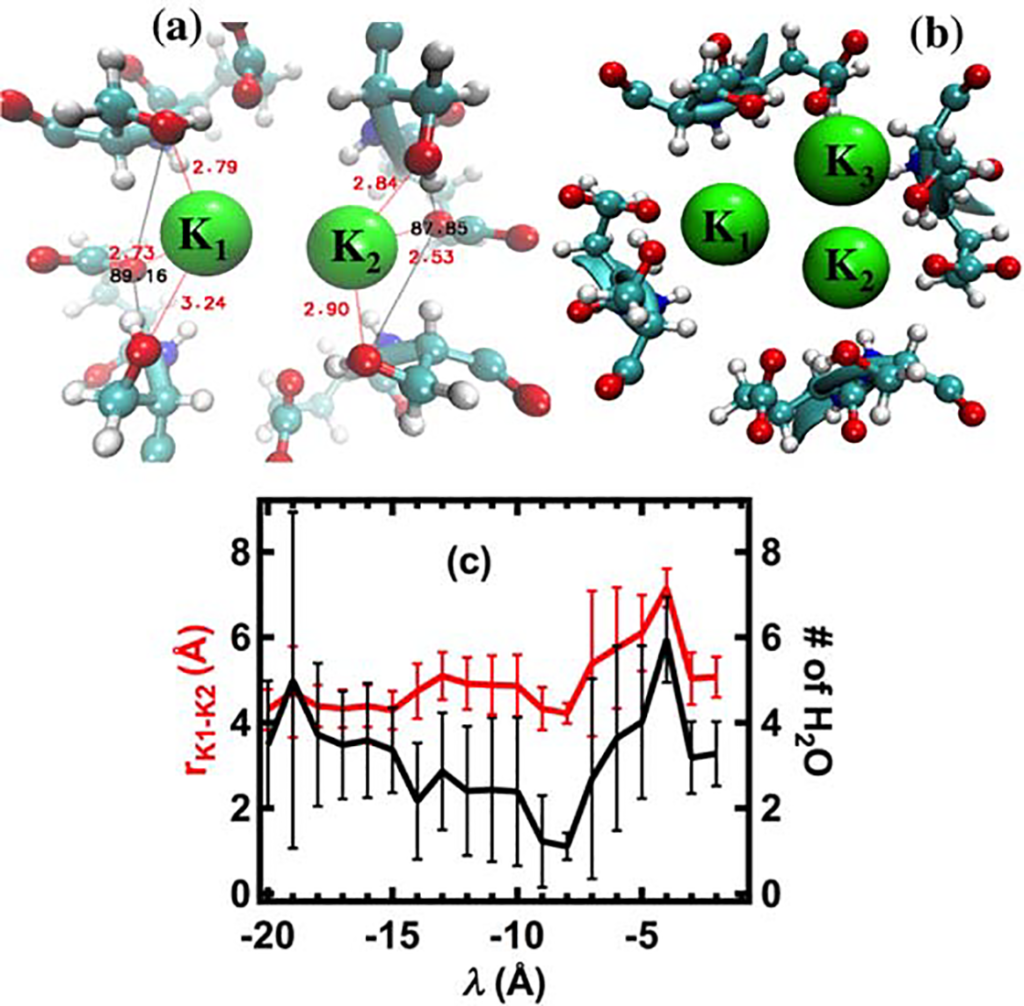
Title
The new editor comes with a handful of default blocks such as paragraph, image, gallery, and more, to help you create better standard posts and pages.
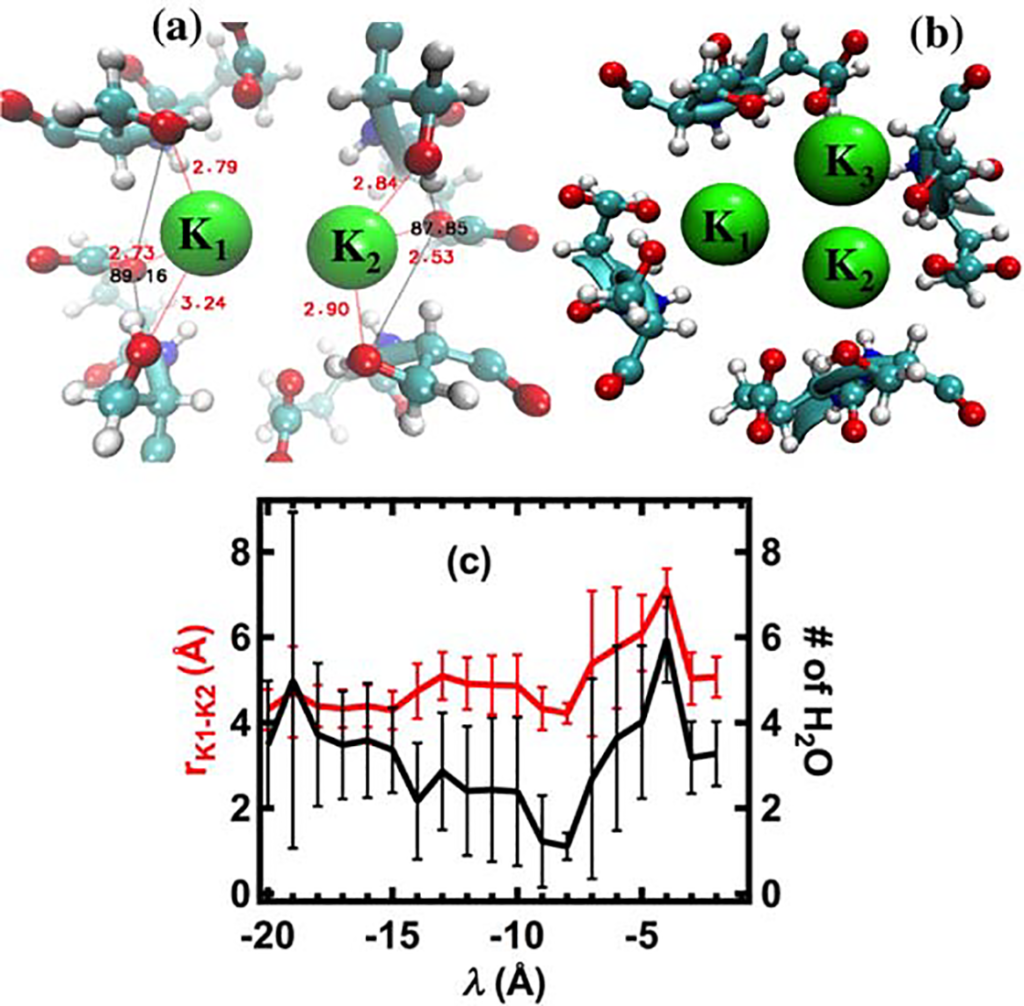
Title
The new editor comes with a handful of default blocks such as paragraph, image, gallery, and more, to help you create better standard posts and pages.
In-Silico Safety Pharmacology
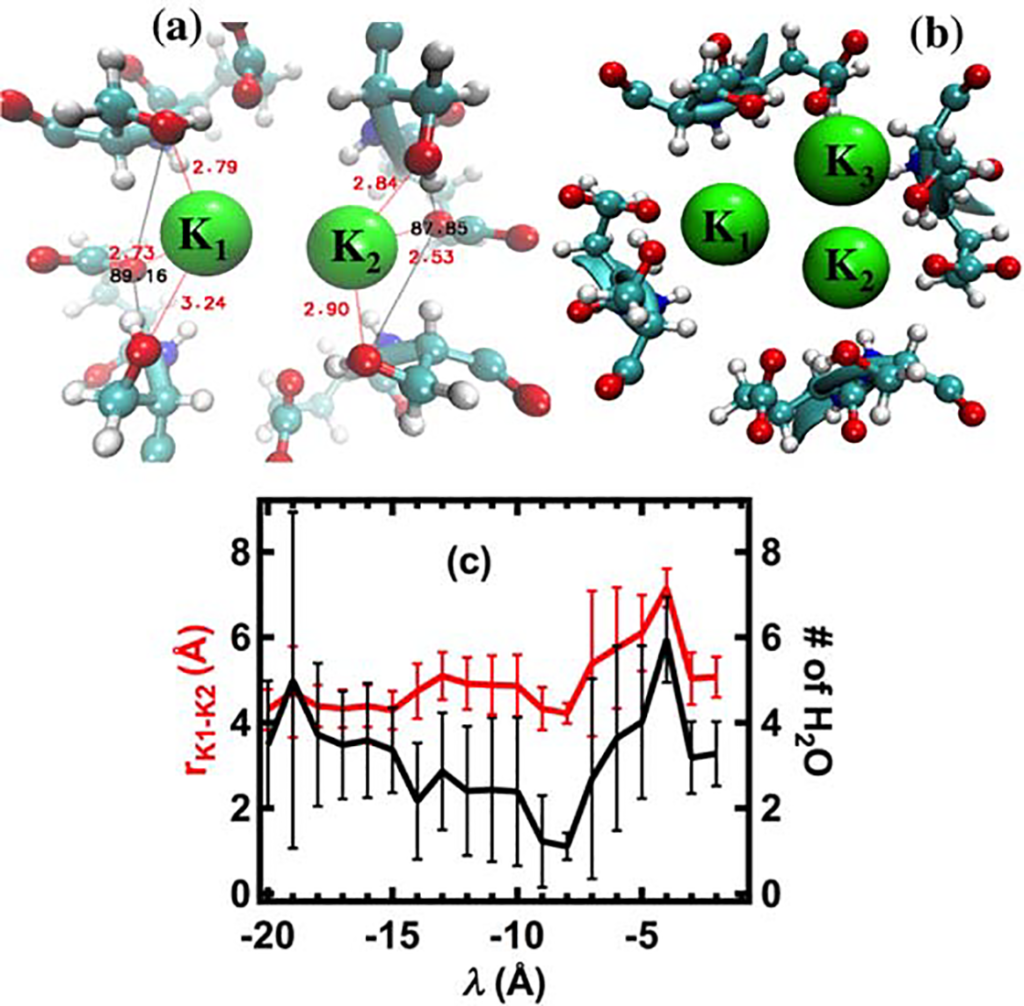
Title
The new editor comes with a handful of default blocks such as paragraph, image, gallery, and more, to help you create better standard posts and pages.
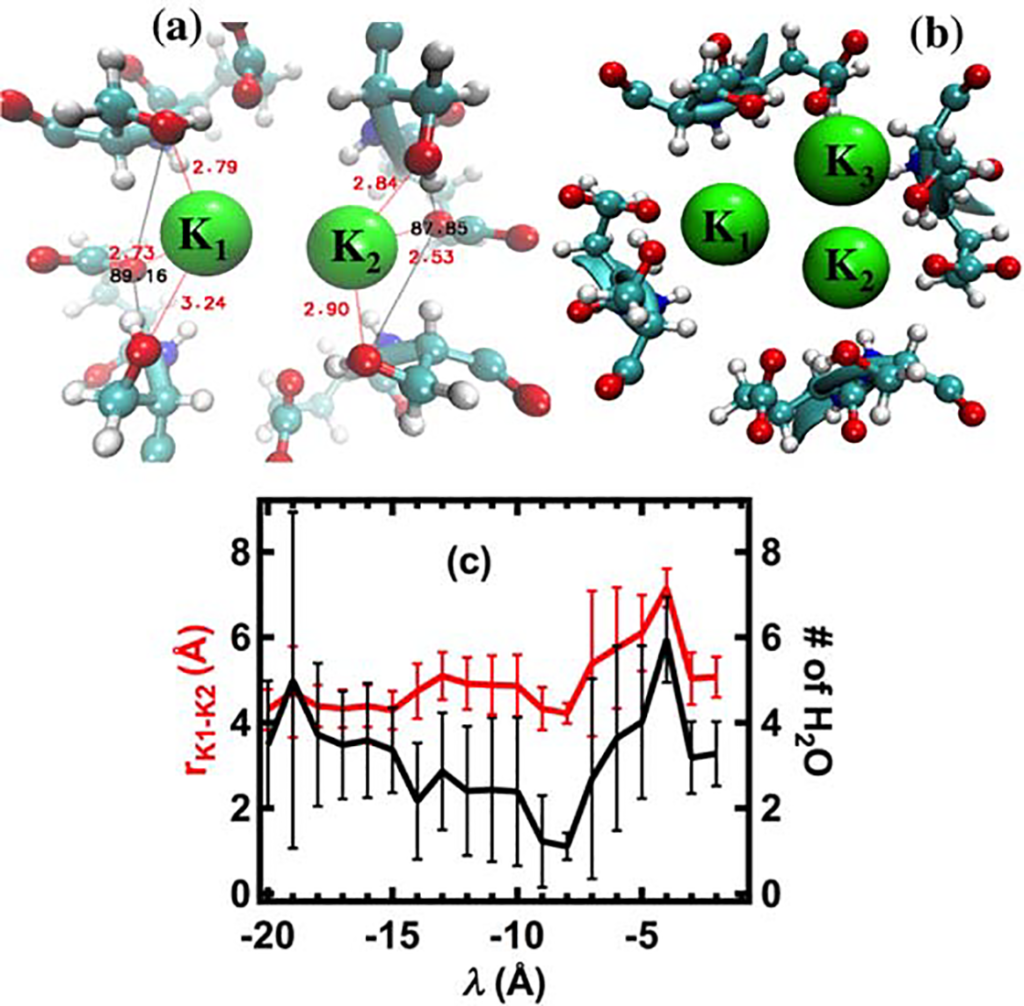
Title
The new editor comes with a handful of default blocks such as paragraph, image, gallery, and more, to help you create better standard posts and pages.
Big Data in Biology: Machine Learning for Omics and Toxicology
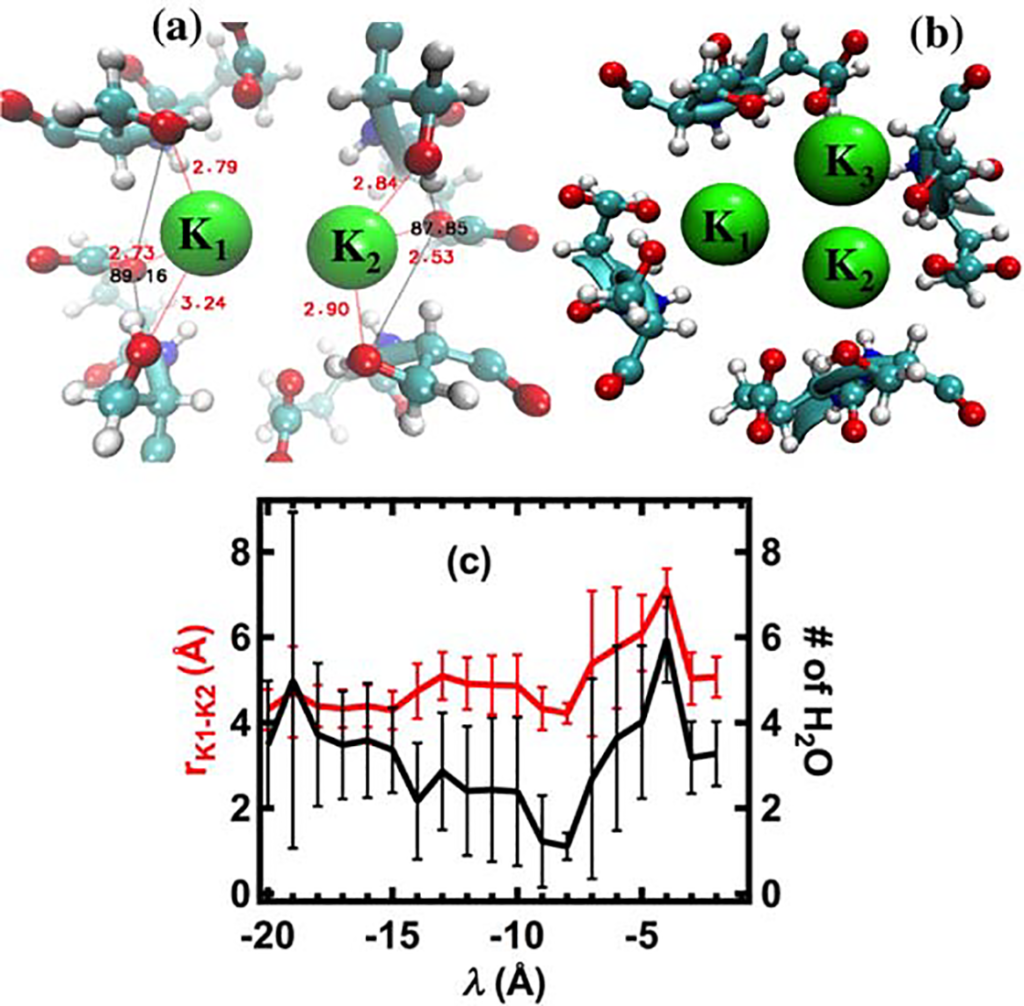
Title
The new editor comes with a handful of default blocks such as paragraph, image, gallery, and more, to help you create better standard posts and pages.
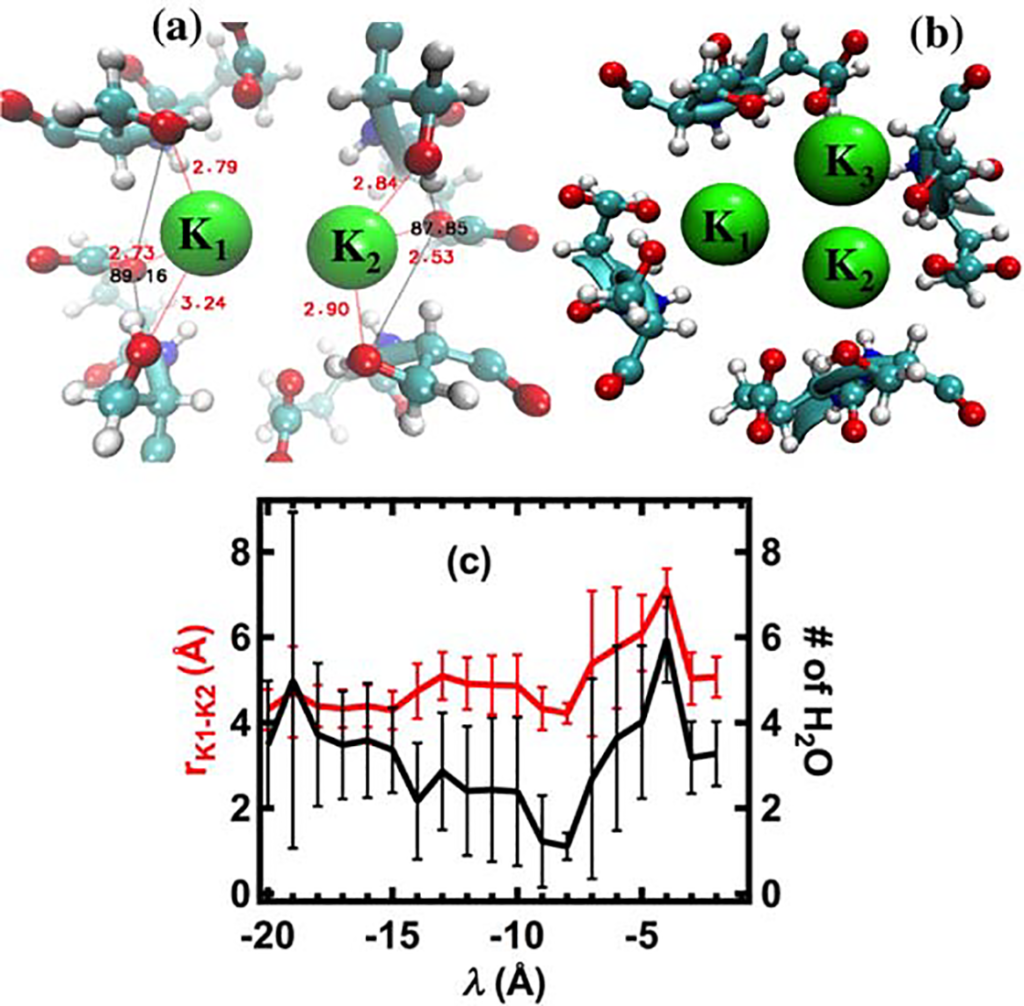
Title
The new editor comes with a handful of default blocks such as paragraph, image, gallery, and more, to help you create better standard posts and pages.